UC San Francisco leadership is determined to become a national leader in medical AI, which offers both unprecedented opportunities and daunting challenges. UCSF researchers hope to roll out increasingly sophisticated and clinically relevant AI algorithms over the next few years, an effort that includes deciding on appropriate therapeutic targets, discerning the most trustworthy approaches for the prospective evaluation of AI algorithms, and ensuring that AI minimizes inequities in care quality and delivery rather than amplifying them. If that sounds like a lot, it’s because it is.
The Department of Epidemiology and Biostatistics will contribute significantly to UCSF’s plan to deliver better care to a wide spectrum of patients using AI algorithms, according to department chair Mark Pletcher, MD, MPH.
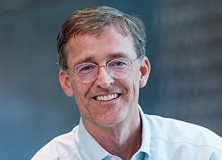
“AI can potentially help clinicians a lot with various aspects of their work,” he said. Pletcher has a keen interest in research methodology and clinical decision-making, and, for a decade, has also directed the informatics program at UCSF’s Clinical and Translational Sciences Institute (CTSI). CTSI aims to help researchers use the University’s electronic health records system, APeX, for research and innovation in clinical care.
Generative AI programs (e.g., ChatGPT) fall under the category of machine learning and are trained on extensive data sets, which they use to generate text, images, and other content. “GenAI knows a lot about medical care and physiology,” Pletcher continued. “But to be useful for clinicians, it needs to be introduced into their workflows with careful design – with different forms of input and output than a simple chat interface – so that it efficiently solves a problem for the clinician. It’s more challenging to come up with useful clinical tools than you might think.”
Pletcher emphasized that it’s crucial to prospectively assess AI algorithms long-term. “We’ve been working out ways to evaluate how well these systems work when we implement them in health care delivery,” he said. “That includes embedding randomized trials in our health system, and carefully experimenting with what works for improving quality of care.”
One weakness in existing AI systems is their tendency to “hallucinate” – inventing citations and facts out of thin air in a process that computer scientists don’t yet fully understand. It isn’t hard to imagine how such a human-sounding glitch could negatively affect medical care.
“We have to make sure the programs aren’t giving erroneous suggestions and causing harm,” Pletcher acknowledged. “And on a more subtle level, we want to check for biases based on existing data, so that patients who have faced historical and structural disadvantages encounter less of that. What we absolutely cannot do is make care better for the people who are already getting better care, while making it worse for those who are already vulnerable.”
Yulin Hswen, ScD, MPH, focuses a lot of her attention on health care equity and democratization. Hswen, an assistant professor in the Department of Epidemiology and Biostatistics as well as at UCSF’s Bakar Computational Health Institute, is also on the faculty of the Computational Precision Health Program, managed jointly with UC Berkeley, and an associate editor in AI and medicine for JAMA and the JAMA Network.
Hswen emphasized that biases that sometimes appear in AI algorithms aren’t due to the machinations of some nefarious computer “mind”; rather, they originate from real-world human prejudices that are then echoed in the data.
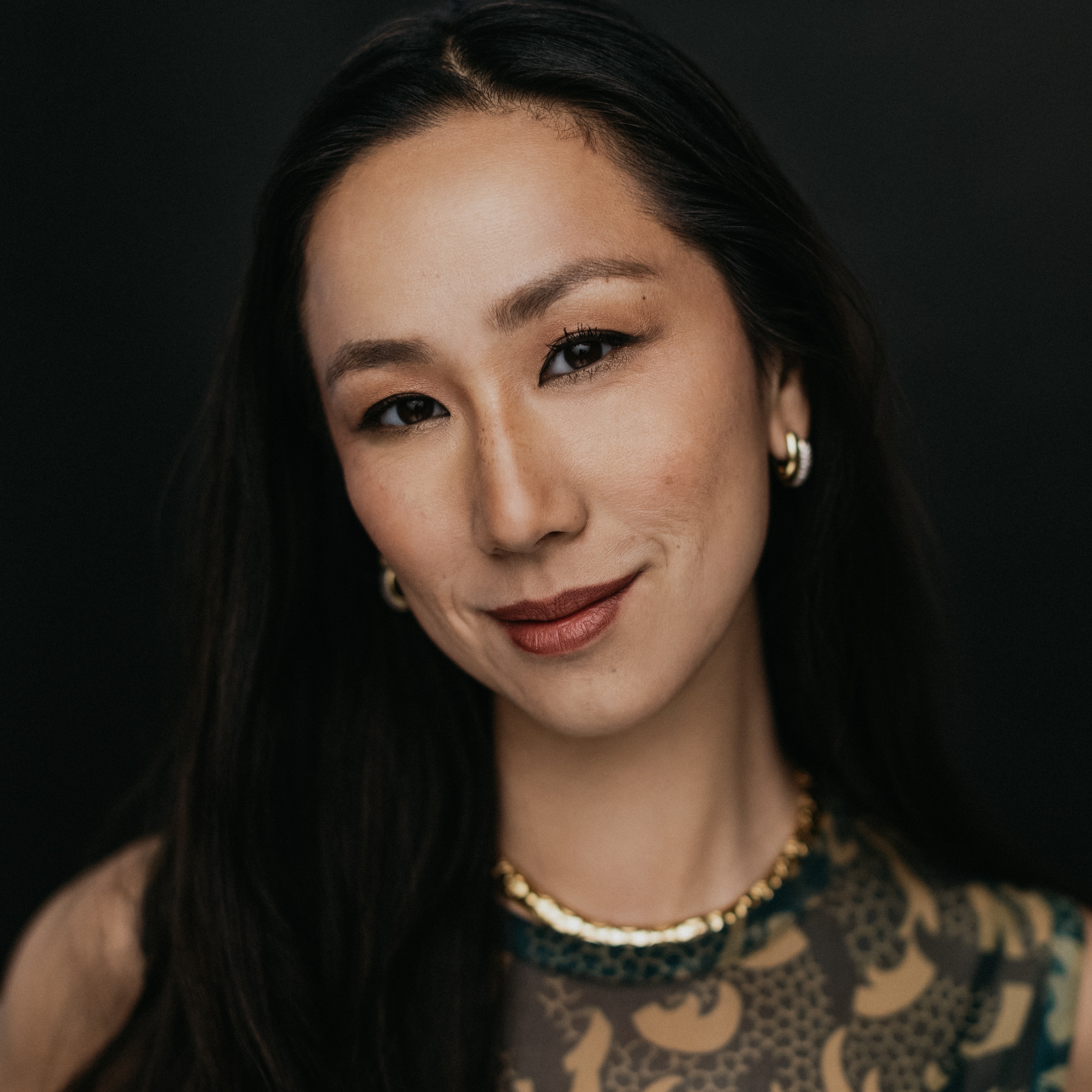
“AI reflects the quality of the data it uses,” she explained. “It replicates human biases. So, we need to start by teaching humans to be more fair and equitable. When the data are less biased, AI will be less biased.”
Hswen advocates for diversity in datasets, which would allow researchers to explore analyses that take into account social determinants of health, including race, ethnicity, gender, and socioeconomic status. She points out that relying solely on average data can overlook the unique needs of underserved populations, underscoring that what benefits the majority may not serve the needs of everyone.
“AI bias can come from training a model on the average and dropping out everything else,” she explained. “So just because the overall ‘average’ patient does better on a certain treatment protocol doesn’t guarantee that other groups aren’t actually faring worse.” A classic example of such bias is that, historically, clinical trials tended to enroll more men than women and did not appropriately analyze sex-specific responses, thus hindering the ability to understand women’s reactions to medications. Such exclusion of certain populations can result in less effective diagnostic and treatment strategies, inadequate reporting of side effects, and ultimately higher rates of morbidity and mortality among underrepresented groups.
Hswen is encouraged by UCSF’s collaboration with five other UC medical centers in collecting data on 9.1 million fairly diverse patients, so far spanning 11 years.
“I’m excited about that eleven-year longitudinal data,” she said. “Most clinical trials are followed for only one or two years. But with large, longitudinal databases like this, you can follow patients longer and potentially gain a fuller perspective on what occurs. Another advantage is that the diversity among those nine million patients enables stratification and examination of various subpopulations and groups in detail.”
Hswen nevertheless worries that, in underserved regions and populations, AI may not be implemented in a way that enhances the health care provided by human clinicians. Instead of enhancing human-delivered care, that is, AI might simply take its place.
“AI should augment human care, not replace it,” she said. “The best outcome would be to have the same level of care in low-resourced areas that patients receive at larger, wealthier urban academic medical centers.”
A related problem is known as automation bias, which means that even when clinicians are involved, they may start defaulting to whatever the AI algorithm says and stop thinking for themselves. Hswen dubbed this phenomenon “cognitive complacency,” and Mark Pletcher expressed a similar concern.
“An algorithm could be right so often that you just start relying on it and stop doing your own reasoning,” Pletcher said. “I don’t know what the answer is to that. If it turns out to be better than we are, should we just use it and go with it? What kind of guardrails do we put up? We’re still figuring that out.”
Hswen, like Pletcher, is also concerned about how AI algorithms will be evaluated going forward. She noted that drugs, vaccines and similar treatments must be approved by the FDA, and she thinks AI should require similar scrutiny.
“To be deployed, AI algorithms should have to be shown to be effective or better than standard or equivalent care,” Hswen said. “We need standards, testing and validation.”
As it happens, Jean Feng, MS, PhD, specializes in developing and evaluating AI algorithms. Feng, like Hswen, is an assistant professor in the Department of Epidemiology and Biostatistics and the UCSF–UC Berkeley Joint Program in Computational Precision Health. Her expertise lies in biostatistics, machine learning, and computer science, with an eye toward researching the interpretability and reliability of machine learning in health care.
AI algorithms present new methodological challenges for several reasons, she said. They can be difficult to interpret and evaluate; they are dynamic and can lead to feedback loops; they tend to perform better for majority groups than subpopulations; and they may not generalize well, especially if built on relatively old data.
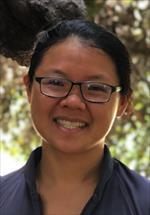
“Half of what I do is think about the math behind algorithms, to be sure they work correctly, reliably and fairly,” Feng continued. “But it’s also important to build predictive models, so you know what problems occur in practice.”
To that end, Feng collaborates with others at UCSF to help build out algorithms, develop monitoring pipelines, and consider experimental and clinical trial designs to evaluate those algorithms.
She is also the data science lead for the predictive analytics team at Priscilla Chan and Mark Zuckerberg San Francisco General Hospital and Trauma Center. In that case, she and her team are building an algorithm to predict the risk that any given patient will be readmitted to the hospital within 30 days after discharge following a previous admission.
“That’s a standard performance measure, and hospitals are financially penalized if patients are readmitted within thirty days,” Feng said. “So ideally we’d like to be able to predict the risk of that.”
Feng and her team are currently focusing on readmission in heart-failure patients, but even with that narrower focus it’s a surprisingly complex problem.
“A lot of things happen both inside and outside of the hospital that are not fully captured by the data in the patient’s electronic health record,” she explained. “We have superficial summaries about your diagnoses, medications and procedures. But then you’re released into the wild, and who knows what happens?”
For Feng, collaboration is the key to eventually solving these thorny issues. She has three clinicians from Zuckerberg San Francisco General on her team, as well as several people in clinical IT who help integrate models into the APeX system and extract data to assist in training algorithms. Team members also regularly discuss priorities with hospital leadership and talk to the clinicians who will likely end up using the algorithms.
“I rely on the expertise of all these people to build out an algorithm that makes sense,” Feng said. “You have to loop in the stakeholders, and you also want to include patients in the process, so they can be more involved in their clinical decision making.
One particularly challenging aspect of the process, according to Feng, is that it’s increasingly clear that algorithms can be – or become – unreliable in mysterious and unpredictable ways.
“They can be a black box,” she admitted. “There are a lot of different ways to evaluate them, and I don’t think any one method has solved the problem yet. Monitoring seems easy but it really isn’t that easy. That’s where a lot of my methodological development has been for the past two years.”
As noted, one of AI’s weaknesses is its indiscriminate incorporation of human bias. It’s built, essentially, to compile and generalize rather than to judge. This quality may become a liability when challenged by complex problems like diagnostics, where judgment is desirable if not essential. Most clinicians know who the best diagnosticians are at their medical center – that is, the doctors to whom patients are referred when no one else can figure out what’s wrong with them. These physicians often have unique traits that include empathy, careful listening, significant clinical experience and expertise, emotional intelligence, and refined intuition. AI algorithms currently have no ability to access or take advantage of such human qualities.
“A computer will never have a human lifetime of experiences,” Mark Pletcher acknowledged. “That experience is what you bring to the table when you’re a doctor taking care of patients, and it’s hard to replace that.”
Though it’s conceivable we might some day try to identify those excellent clinicians and use them to train AI algorithms, this would entail complex issues of its own. Pletcher said that even if you could identify and enlist the doctors who should take the lead in training AI – then somehow have AI learn more from them and augment its spongelike absorption of general data – the system isn’t yet capable of this. AI algorithms aren’t learning from medical reasoning in real time.
“I do think AI will eventually have the capacity to learn in better ways,” he said. “But right now, they just have a big, trained neural network that doesn’t learn new things, but rather applies what it’s learned to new situations, to new prompts and inputs.”
As the Department of Epidemiology and Biostatistics moves AI forward, UCSF researchers and their patients have much to gain. Ideally, progress will lead to algorithms that are safe, transparent, accountable, reliable and fair. It may be a bumpy ride, but the goal holds so much promise that those involved are buckling up and getting ready for the trip.