It’s not surprising that the faculty in the Biostatistics division of the Department of Epidemiology and Biostatistics are sought-after collaborators on campus, given that UCSF is the top public recipient of National Institutes of Health research funding.
“Biostatistics is the language of research—how to design a study, how to analyze the data,” says Chuck McCulloch, division chief. “We’re fortunate that we’re in a university that values our work.”
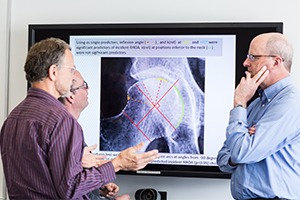
McCulloch and the other dozen biostatistics faculty collaborate on research in areas as diverse as cancer, osteoarthritis, cardiovascular disease, HIV, genetics, mental illness and many, many more.
“There is so much research flowing through UCSF, and virtually every study needs statistics, so there are a lot of opportunities to collaborate,” McCulloch says. “Our biostatistics faculty come to UCSF because they want to be embedded in medical research and do their own research.”
That said, McCulloch points out that faculty are pulled in multiple directions: teaching and consulting as well as collaborating and conducting biostatistics methodology research.
Faculty are expected to contribute to their field and are doing innovative research that advances the discipline of biostatistics. Across the division, the faculty are publishing in the top biostats journals.
Here are just two examples:
John Kornak, who also is leading the development of a data science education program, researches methods to improve data that is derived from images.
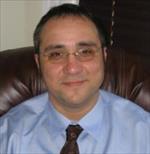
“Many imaging formats are not as clean as you’d hope in order to get the information a researcher or clinician wants. I’m working on statistical methods to enhance images to better detect clinically important information,” Kornak says.
By translating raw images into a different mathematical space, image analysis becomes easier and less expensive, and it can be more targeted to specific clinical problems. Clinicians could use Kornak’s method to detect changes in image properties more quickly, easily and accurately. For example, it might be used to detect brain changes as dementia progresses or tumor responses to breast cancer treatment.
Chiung-Yu Huang develops methods to analyze data that consists of times to events, called survival analysis, such as time spent disease-free after cancer treatment. Her specialty is “recurrent event data,” when the times to event can happen repeatedly, such as times between admission to emergency rooms for children with asthma.
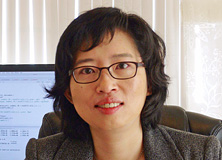
Survival analysis is a way to deal with incomplete information, Huang says. For example, if a researcher is conducting a 5-year study of the time from diagnosis to death, it is unlikely that all study participants will die during the follow-up period. Huang’s methodology allows the researcher to use all participant data and make inferences based on this incomplete information.
“I feel like my research helps people solve problems,” Huang says. “If the researcher ignores that data are incomplete, the inference could be wrong and s/he could make a wrong conclusion, such as underestimate deaths.”
As a member of the Helen Diller Family Comprehensive Cancer Center, Huang also helps design studies and analyze data with colleagues there. She recently became lead statistician for the Surgery Department as well.