A new UCSF study will take data gathered from a new machine and crunch it using modern machine learning to help predict which cerebral aneurysms are likely to rupture.
The machine, known as a 4D Flow MRI, can create an image of an aneurysm in three physical dimensions and in the fourth dimension of time, creating a large amount of useful data that reveals forces exerting pressure on the aneurysm.
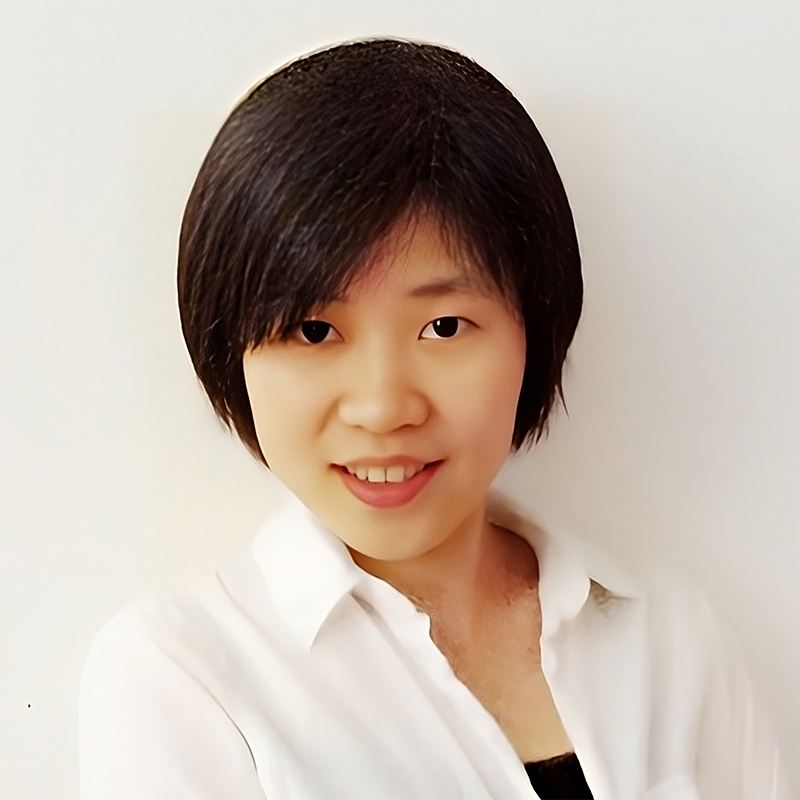
Fei Jiang, PhD, MS, an associate professor of epidemiology and biostatistics at UCSF, was awarded more than $500,000 in a National Institutes of Health R01 grant to predict the growth of aneurysms.
Only about 6 percent of people develop cerebral aneurysms, which, in most cases, rarely cause symptoms and do not require invasive treatment. These so-called unruptured intracranial aneurysms (UIAs), however, can cause great damage and even death if they rupture. Jiang estimated about one-third of aneurysms rupture, but noted it is very difficult to predict which ones.
Doctors don’t want to pre-emptively remove all aneurysms because the procedure is invasive and could cause severe damage. Successfully predicting which aneurysms are likely to rupture and then removing those could prevent serious injury and death.
Yet the current standard, Jiang said, is to measure the size of the aneurysm, which is not very accurate. The risk of rupture also depends on the pressure around the aneurysm, Jiang said.
A New Machine
“The grant is about using a novel image modality called 4D Flow,” Jiang said, referring to a type of MRI that reveals the flow inside the UIA’s blood vessels—what is known as the hemodynamic features of UIAs.
Recent evaluations of UIAs using 4D Flow suggest certain hemodynamic variables may greatly impact aneurysm growth or rupture. But they’re not ready for doctors to apply them in making clinical decisions because scientists have not yet proven their relationship to UIA growth.
“We propose 4D Flow as a non-invasive method to measure the blood flow around aneurysms so that we can extract some features from the blood flow to say whether these features are associated with the growth trajectory of the aneurysms,” Jiang said.
Jiang said doctors will ultimately have a great deal of data about each individual aneurysm—its size, how it’s changed over time, and the pressure inside and outside the aneurysm.
Plugging that large volume of data from 4D Flow into a machine-learning model may yield enough information to decide whether the aneurysm poses a risk of rupture.
Learning from the Data
“If you just look at the data, nobody actually understands what's going on with the patient,” said Jiang, a data scientist. “So we have proposed a testing pipeline to extract the information from the data and then display it in a better visualization, so that the physician knows the pressure at each point.”
“The physician can look at the trajectory to decide whether it's a stable aneurysm that we don't need to worry about or one that we need to have an intervention and remove,” she said. If the aneurysm does need to come out, the data could reveal the best time for the surgery.
“If, say, a person is 80 years old, and the prediction is that the aneurysm will grow large enough to rupture in 10 years, the physician will probably decide not to remove it because removing it may cause cognitive impairment,” Jiang said. “There are pros and cons in removing those aneurysms.”
The project originated in Jiang’s collaboration with UCSF radiologists David Saloner, PhD, who is chief of radiology research at the San Francisco Veterans Affairs Medical Center; Dimitrios Mitsouras, PhD, professor of radiology and biomedical imaging at UCSF anda medical physicist at the VA; and Matthew Amans, MD, a professor of radiology and neurological surgery.
Initially, the researchers will study about 150 people with unruptured aneurysms. If the project succeeds, a follow-up study will use a much larger cohort, Jiang said.
Combining Methods
The ability to use machine learning models to help predict what an aneurysm might do is exciting, Jiang said, but she’s most inspired by the new 4D Flow technology developed by GE that makes gathering the raw data possible. “Without this new technology, we wouldn’t even have a way to gather the information to develop the algorithms,” she said.
One key to the project’s success will be combining the 4D Flow data with computational flow dynamics—essentially, a computer simulation of the blood vessels that help fill in the gaps of what the doctors know. The simulation can have a high resolution, Jiang said, but may not be accurate; the 4D Flow data is more accurate, but it often has a low resolution because doctors don’t want to make vulnerable patients endure long, uncomfortable tests.
Once the data is gathered, combined with the simulation, and analyzed, the next critical step is to make it understandable to clinicians. “The visualization of this type of data is in high demand in our university right now,” she said. “One of our goals is, once we finish the grant, we want to have some kind of platform for the clinician to visualize the data.”